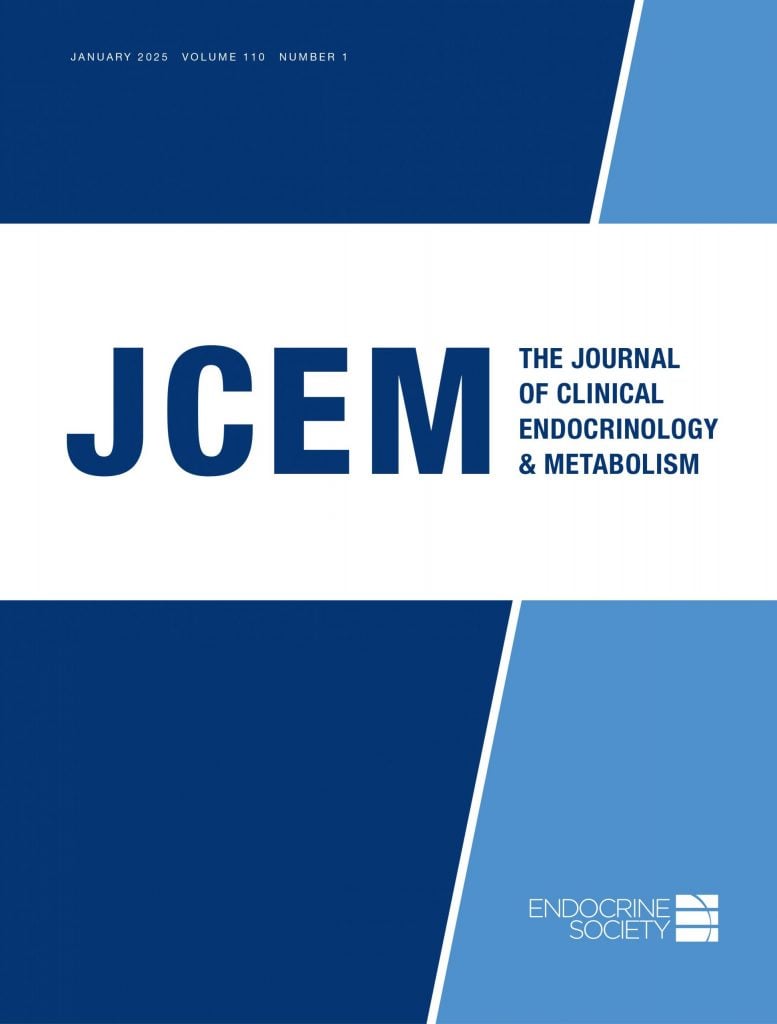
Doctor AI will see you now — eventually. A new study from The Journal of Clinical Endocrinology & Metabolism has demonstrated how artificial intelligence (AI) can enhance the accuracy of diagnosing Cushing’s syndrome (CS), a rare but serious endocrine disorder caused by excessive cortisol production.
Cushing’s syndrome is characterized by a broad spectrum of symptoms, including weight gain, hypertension, diabetes, and osteoporosis, many of which overlap with more common conditions such as metabolic syndrome. Because of this, misdiagnosis or delayed diagnosis is common, making early and accurate detection crucial. The Endocrine Society guidelines suggest screening for CS in adult patients with unusual features for their age (such as easy bruising, moon face, purple stretch marks, and unusual fat pads), as well as in individuals with those multiple or progressive chief complaints that best discriminate CS. These guidelines recommend three key screening tests to detect hypercortisolism: the 1-mg overnight dexamethasone suppression test (DST), which measures how cortisol levels respond to a steroid dose; urinary free cortisol (UFC), which evaluates cortisol excretion over 24 hours; and late-night salivary cortisol (LNSC), which detects abnormal cortisol rhythm (cortisol levels should be low at nighttime).
While traditional screening tests remain effective, this study highlights the growing role of AI in endocrinology. By integrating machine learning and clinical expertise, doctors can improve early detection and accurate diagnosis of conditions like Cushing’s syndrome, leading to better patient outcomes and more personalized treatment strategies.
The current study, “Clinical and Biochemical Data for the Diagnosis of Endogenous Hypercortisolism: The ‘Cushingomic’ Approach,” which analyzed 655 patients, found that traditional screening tests remain highly effective, but integrating AI-based analysis can further refine diagnosis, reducing false positives and improving clinical decision-making. To refine the diagnosis of Cushing’s syndrome, the study authors applied principal component analysis, K-means clustering, and neural network modeling. These AI-driven techniques helped analyze not just cortisol levels, but also patient comorbidities, signs, and symptoms to create a more comprehensive diagnostic approach.
The study confirmed that DST and UFC were slightly superior to LNSC in diagnostic accuracy. However, patients with adrenal incidentalomas (AI) who had mild cortisol overproduction required a higher DST threshold (196 nmol/L) to distinguish them from true Cushing’s syndrome cases. The most groundbreaking finding was the performance of a neural network model, which combined cortisol screening tests with clinical presentation data to predict CS with 99% precision and 86% accuracy. This suggests that AI-assisted analysis could help streamline diagnosis, guide treatment decisions, and reduce the burden of unnecessary testing on patients.
The team lead by Filippo Ceccato, MD, PhD, grouped patients without CS into three distinct clusters: 1. Older patients with osteoporosis and abnormal screening tests, 2. Individuals with hypertension and metabolic conditions (obesity, diabetes), whose symptoms could mimic CS, and 3. Younger individuals with a low likelihood of overt CS. By distinguishing these groups, doctors can avoid unnecessary testing and false-positive results in patients who do not actually have CS.
While traditional screening tests remain effective, this study highlights the growing role of AI in endocrinology. By integrating machine learning and clinical expertise, doctors can improve early detection and accurate diagnosis of conditions like Cushing’s syndrome, leading to better patient outcomes and more personalized treatment strategies. As AI-driven models like the ‘Cushingomic’ approach become more refined, they could transform how endocrine disorders are diagnosed, making healthcare more precise, efficient, and accessible.